Program | Perspective Talks
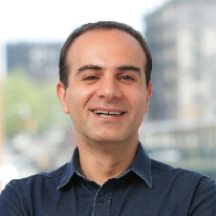
Speaker
Nima Mesgarani, Electrical Engineering Department, Columbia
University in the City of New York
Webpage
Title
Speech Processing in the Human Brain Meets Deep Learning
Abstract
Speech processing technologies have seen tremendous progress since the advent of deep learning, where the most challenging problems no longer seem out of reach. In parallel, deep learning has advanced the state-of-the-art in processing the neural signals to speech in the human brain. This talk reports progress in three important areas of research: I) Decoding (reconstructing) speech from the human auditory cortex to establish a direct interface with the brain. Such an interface not only can restore communication for paralyzed patients, but also has the potential to transform human-computer interaction technologies, II) Auditory Attention Decoding, which aims to create a mind-controlled hearing aid that can track the brain-waves of a listener to identify and amplify the voice of the attended speaker in a crowd. Such a device could help hearing-impaired listeners communicate more effortlessly with others in noisy environments, and III) More accurate models of the transformations that the brain applies to speech at different stages of the human auditory pathway. This is achieved by training deep neural networks to learn the mapping from sound to the neural responses. Using a novel method to study the exact function learned by these neural networks has led to new insights on how the human brain processes speech. On the other hand, these new insights motivate distinct computational properties that can be incorporated into the neural network models to better capture the properties of speech processing in the human auditory cortex.
Biography
Nima Mesgarani is an associate professor at Electrical Engineering Department and Mind-Brain-Behavior Institute of Columbia University in the City of New York. He received his Ph.D. from the University of Maryland and was a postdoctoral scholar in Center for Language and Speech Processing at Johns Hopkins University and the Neurosurgery Department of University of California San Francisco. He has been named a Pew Scholar for Innovative Biomedical Research, and has received several distinctions including the National Science Foundation Early Career Award and Kavli Institute for Brain Science Award. His interdisciplinary research combines theoretical and experimental techniques to model the neural mechanisms involved in human speech communication which critically impacts research in modeling speech processing and speech brain-computer interface technologies.
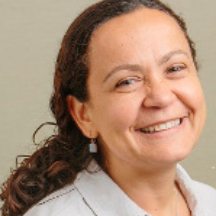
Speaker
Dilek Hakkani-Tur, Research Scientist, Amazon, USA
Webpage
https://scholar.google.com/citations?user=GMcL_9kAAAAJ&hl=en
Title
Deep Learning Based Situated Goal-oriented Dialogue Systems
Abstract
Interacting with machines in natural language has been a holy grail since the beginning of computers. Given the difficulty of understanding natural language, only in the past couple of decades, we started seeing real user applications for targeted/limited domains. More recently, advances in deep learning based approaches enabled exciting new research frontiers for end-to-end goal-oriented conversational systems. In this talk, I’ll review end-to-end dialogue systems research, with components for situated language understanding, dialogue state tracking, policy, and language generation. The talk will highlight novel approaches where dialogue is viewed as a collaborative game between a user and an agent in the presence of visual information, and will aim to summarize challenges for future research.
Biography
Dilek is a research scientist at Amazon and has previously held research scientist positions at Google, Microsoft Research, ICSI, and AT&T Labs – Research. She is a fellow of the IEEE and of ISCA. Her research interests include conversational AI, natural language and speech processing, spoken dialogue systems, and machine learning for language processing.
Speaker
Sriram Ganapathy, Department of Electrical Engineering, Indian Institute
of Science, Bangalore, India
Webpage
http://leap.ee.iisc.ac.in/sriram/
Title
Speaker and Language Recognition -- From Laboratory Technologies to the Wild
Abstract
Detecting the paralinguistic components of speech like speaker and language is of substantial interest for many commercial, surveillance and security applications. The problem is at least three decades old with some of the early techniques based on simple Gaussian mixture models. A significant advancement in this area came about a decade ago with the advent of joint factor analysis and i-vector models. The last couple of years have seen further breakthroughs with deep embeddings and end-to-end models based on deep learning. With these improvements in modeling speaker and language, the application of the technology has also moved from clean controlled speech data to telephone channel recordings, far-field microphones and more recently to multi-speaker conversations in the wild. In the talk, I will provide a prospective view of the broad research directions in the field of speaker and language recognition. I will also highlight some of the recent advancements from our work on hierarchical end-to-end approaches with relevance modeling.
Biography
Sriram Ganapathy is a faculty member at the Department of Electrical Engineering, Indian Institute of Science, Bangalore. Previously, he was a research staff member at the IBM Watson Research Center. He received his PhD from the Center of Language and Speech Processing, Johns Hopkins University. His research interests include signal processing and machine learning applied to speech recognition, speaker recognition and auditory neuroscience. He is a member of the ISCA and a senior member of the IEEE.
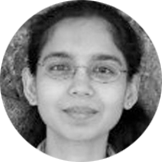
Speaker
Bhuvana Ramabhadran, Google Inc., USA
Webpage
https://scholar.google.com/citations?user=jecEO0EAAAAJ&hl=en
Title
Open Problems in Speech Recognition
Abstract
In this talk, I will focus on the evolution of ideas in speech recognition over the last couple of decades, with emphasis on the key breakthroughs over the last ten years, its impact across spoken language processing in several languages, recent trends and open challenges that remain to be addressed. One such breakthrough is the use of several neural network model variants, which has had an enormous impact on the performance of state-of-the-art large vocabulary speech recognition systems. They have also had impact on keyword search which is the task of localizing an orthographic query in a speech corpus, and is typically performed through analysis of automatic speech recognition (ASR). Using the recently concluded IARPA funded Babel program as an example of a well-benchmarked task that focussed on the rapid development of speech recognition capability for keyword search in a previously unstudied language, I will present the successes and challenges that persist with limited amounts of transcription. Interpreting and understanding the hidden representations of various models remains a challenge today. I will also discuss current research taking advantage of such interpretations to improve robustness to noisy environments, speaker/domain adaptation algorithms, and dialects/accents. I will conclude with relevant metrics to measure speech recognition performance today that include and ignore the bigger picture of end to end user experience.
Biography
Bhuvana Ramabhadran (IEEE Fellow, 2017, ISCA Fellow 2017) currently leads a team of researchers at Google, focussing on multilingual speech recognition and synthesis. Previously, she was a Distinguished Research Staff Member and Manager in IBM Research AI, at the IBM T. J. Watson Research Center, Yorktown Heights, NY, USA, where she led a team of researchers in the Speech Technologies Group and coordinated activities across IBM's worldwide laboratories in the areas of speech recognition, synthesis, and spoken term detection. She was the elected Chair of the IEEE SLTC (2014–2016), Area Chair for ICASSP (2011–2018) and Interspeech (2012–2016), was on the editorial board of the IEEE Transactions on Audio, Speech, and Language Processing (2011–2015), and is currently an ISCA board member. She has served on the editorial board of T-ASLP (2012-2016), technical area chair for ICASSP (2011-2017), Interspeech (2012, 2014-2016), and was one of the lead organizers and technical chair of IEEE ASRU 2011, She has given tutorial and keynote presentations at several international conferences and served as an adjunct professor in Columbia University, where she co-taught a course in speech recognition. She has published over 150 papers and been granted over 40 U.S. patents. She was named a Master Inventor twice by IBM. She is a reviewer for ICASSP, Inerspeech, NAACL, ACL, EMNLP and serves on student dissertation committees and NSF review panels. Her research interests include speech recognition and synthesis algorithms, statistical modeling, signal processing, and machine learning.